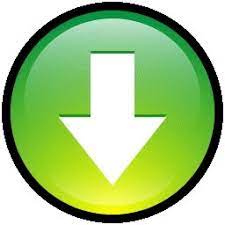
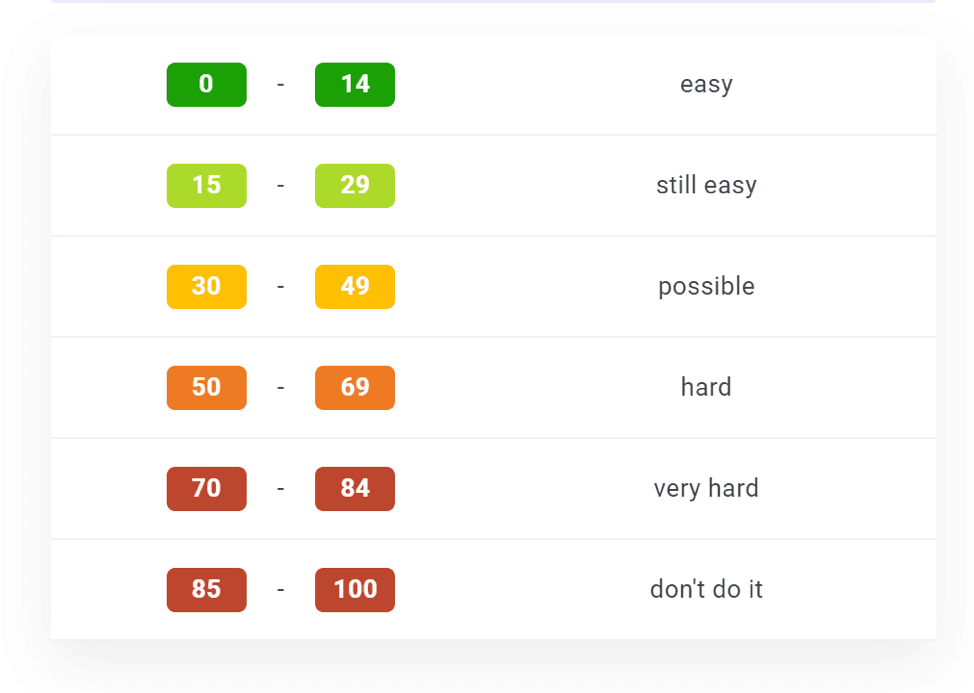
New to crypto? You can find a great overview about block-chain technology, cryptocurrency and how they are related to Satoshi Nakamoto’s innovation. Users often generate a new address for every transaction to help stay anonymous.In this post, we look at correlation of the cryptocurrency market, using Python, to analyze and visualize how these markets have been evolving, and provide a base framework for deeper analysis. This doesn’t necessarily mean the inputs came from different people though. Large transactions may feature many addresses combining their resources, which could explain the number of blue input nodes linked to each. From the purple time bar below the chart, the large peak shows us they happened within one second of each other. In this example, the transactions transferred two large sums of money totaling US $72,000 to the same shared address. Unusual connections in a network are worth exploring. KeyLines displays the additional information we need: Click a node and KeyLines sends a Cypher query to the Neo4j database requesting more information:Ī('B', str(tx.btc),' / ','$', str(tx.usd)),Ī(tx.dt, "dd MMMM yyyy HH:mm") By keeping things simple and avoiding labels, we don’t clutter the chart with too much detail upfront.īut what if it’s the details you’re after? Using clever node sizing, right away you can see the value of one transaction was far greater than the other.
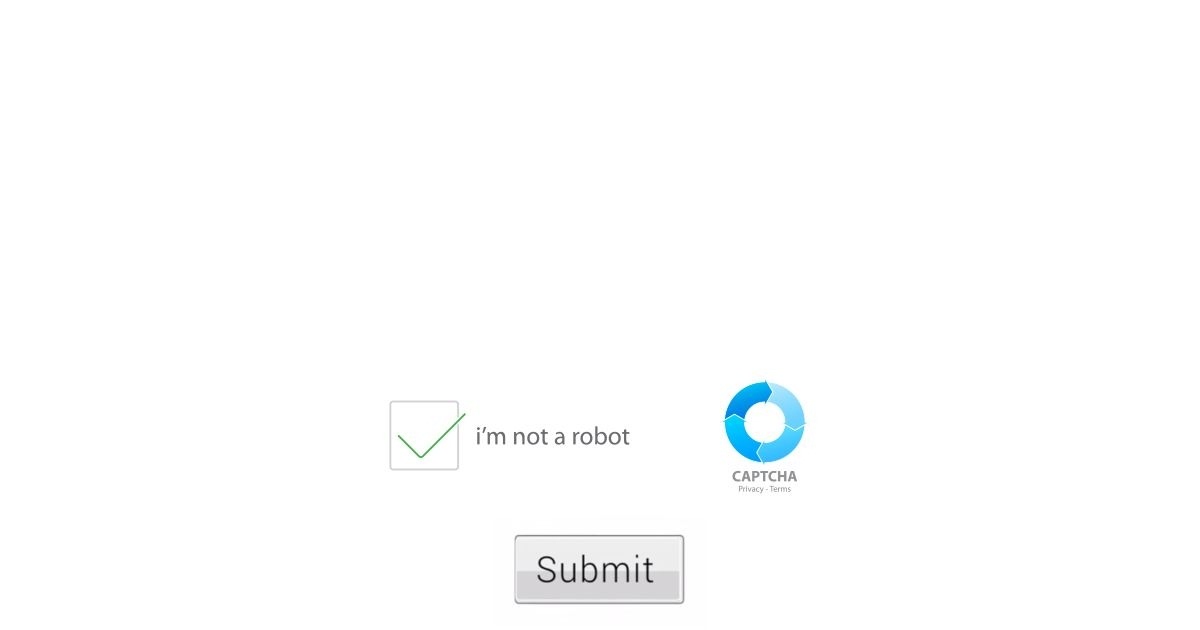
The example on the right shows a standard transaction where bitcoins moved from one address to two others. This typically means there was change owed from the original payment. In the example on the left, the transaction input and output share the same address, so the node is blue and orange. Understanding the nature of Bitcoin transactions helps fraud analysts identify patterns more easily. We took block 611900 from ’s API and followed this great guide to import it into a Neo4j graph database.įinally, we visualized it using KeyLines, our graph visualization toolkit for JavaScript developers.

Here’s a chart of Bitcoin transactions over a six-minute period. When you need to understand a network this huge, Neo4j’s highly scalable graph database and our powerful graph visualization toolkit technology complement each other perfectly. How do link analysts understand Bitcoin transactions? What patterns of behavior must they look out for? From money laundering to monetizing ransomware, buying illegal goods to fraud scams, criminals use it as a secure, low-cost anonymous way to transfer funds quickly and easily.
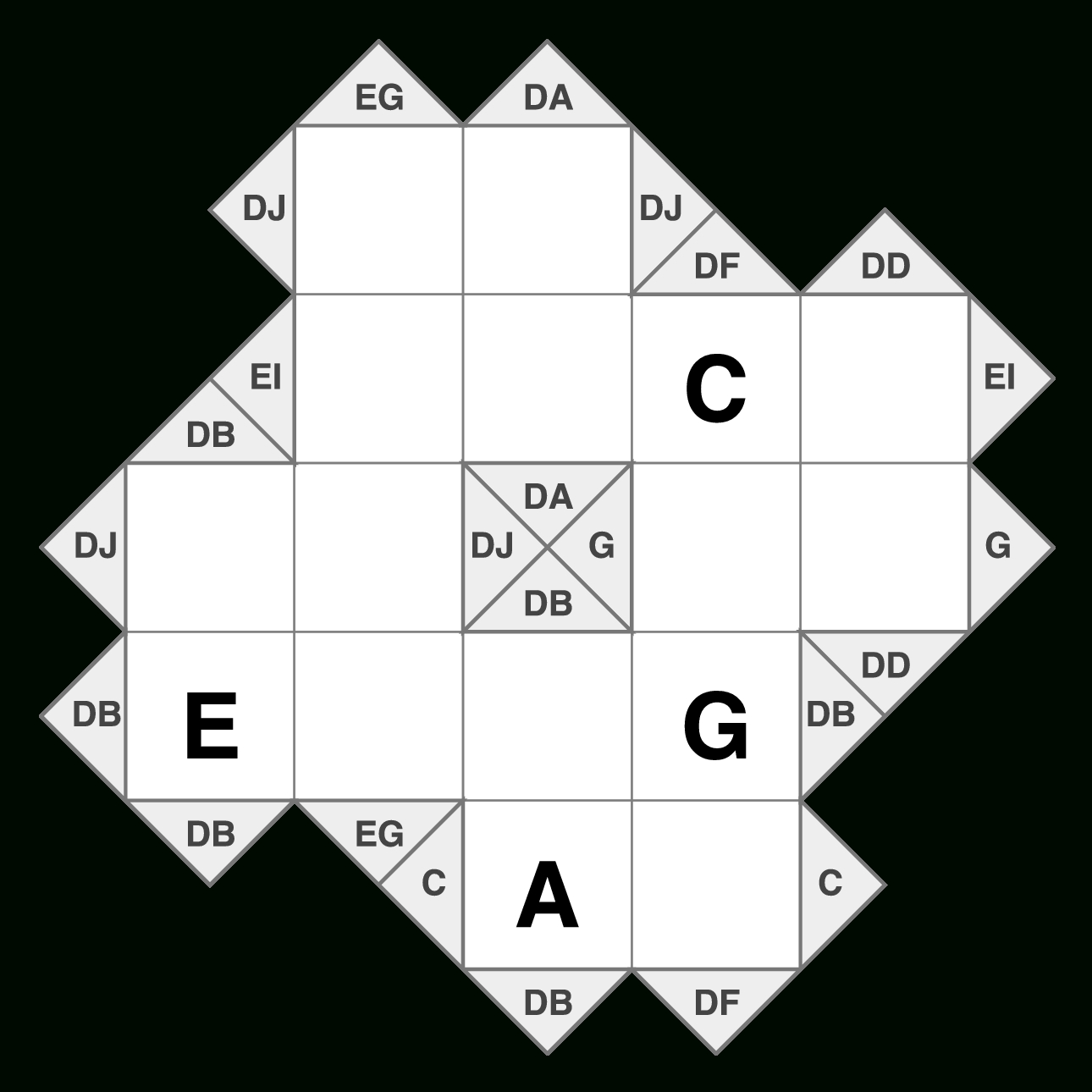
There’s a serious reason for understanding Bitcoin data. How can we understand it better? What does a typical transaction look like compared to an unusual one? How do we avoid getting lost in the giant wall of blocks? If Bitcoin Is a Graph, We Can Visualize Itīitcoin data contains a vast and complex network of connections. The entire dataset – right back to the very first transaction – is available to all.īut making it available is one thing. It shares details of each block with every other Bitcoin user. To stop other users from questioning the validity of a transaction, Bitcoin uses blockchain to maintain and protect the correct order of transactions in separate blocks. Graphs are everywhere, even inside Bitcoin’s public ledger.
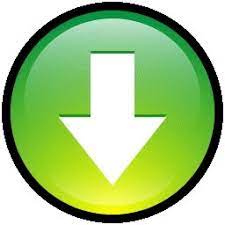